From fraud detection systems that adapt in real time, to investment platforms that learn investor behavior, AI is quietly reshaping the way money moves, decisions are made, and risks are managed.
Global investment in AI across financial services is growing fast. According to IDC, AI is projected to contribute over $22 trillion to the global economy by 2030 - highlighting the scale and momentum behind AI-driven transformation. That kind of momentum isn’t just about AI transformation. It’s about survival in a digital economy where speed, accuracy, and insight drive everything.
In the last decade, Aubergine has worked closely with partners across banks, fintechs, and regulators while building digital products. The shift we see is clear. AI works best not as a side project, but when it’s embedded into the fabric of how things are designed and developed. That’s when you start seeing real returns with less manual oversight, fewer errors, and more intelligent decisions.
This article breaks down the four ways AI is reshaping the future of finance. We’ll also look at the latest research, what industry leaders are saying, and where all this is headed. The goal is to help financial professionals not just keep up, but lead the change.
Four pillars of AI transforming the financial industry
Today, AI is not a feature, but a system. In other words, it’s a collection of powerful capabilities that solve diverse kinds of problems. In finance, four types of AI are leading the way and each has its own strengths and is already changing how institutions work at a core level.
Anomaly AI
The FBI and FTC both reported a sharp increase in financial fraud over the last three years. In the US alone, internet fraud losses exceeded $12.5 billion in 2023, marking a 22% increase from the previous year, according to the FBI’s Internet Crime Report.
Today, it hides in real-time transaction flows, complex identity patterns, and cross-border activity that moves faster than any compliance team can track manually. This is where anomaly detection AI steps in.
At its core, this type of AI is trained to find the unusual and it doesn’t rely on fixed rules. It learns from normal behavior and flags anything that doesn’t fit. For example, a sudden spike in transaction volume or a login from two locations within minutes is enough for an AI system to detect a pattern anomaly and act accordingly.
This is critical because traditional systems generate a high number of false positives. AI understands this context to help sort the signal from the noise. For example, if a user makes a large transaction during a known travel window, the system can learn that pattern and avoid raising an alert.
Banks that use AI for anomaly detection are reducing false positives by up to 60 percent. That’s a massive operational win when teams spend less time chasing false alarms and more time responding to real threats.
Anomaly detection isn’t just about security - it’s about trust. When you can catch fraud early and quietly, customers feel protected and that’s good for business.
Classification AI
Financial institutions are inundated with vast amounts of unstructured data like transaction records, customer interactions, market news, social media sentiments, etc. Making sense of this data is crucial for informed decision-making and personalized customer experiences. This is where Classification AI is transforming finance.
Classification AI involves algorithms that categorize data into predefined classes or groups. In finance, this means sorting customers based on behavior, segmenting transactions by type, or identifying documents by content. By automating these classifications, institutions can streamline operations and enhance decision-making processes.
Applications in Finance
Customer segmentation.
By analyzing customer data, banks can classify clients into segments based on spending habits, risk profiles, or product preferences. This enables targeted marketing and personalized service offerings.
Fraud detection.
Machine learning algorithms analyze patterns in transaction data to detect anomalies that may indicate fraudulent activity.
Transactions can be classified as legitimate or suspicious, enabling real-time fraud prevention measures.
Credit risk assessment.
Classification models evaluate borrower information to predict the likelihood of default, allowing for more accurate lending decisions.
A UK high street bank collaborated with Kortical to enhance its credit risk assessment process. By integrating machine learning models, the bank aimed to improve the prediction of customer defaults compared to traditional credit scoring methods.

The AI model developed was able to identify 83% of bad debts that were previously missed by conventional credit scoring, without increasing the number of loan rejections. This advancement allowed the bank to make more informed lending decisions, reducing potential losses and improving overall portfolio health.
Implementing Classification AI has shown tangible benefits. For instance, banks utilizing AI-driven customer segmentation have reported increased cross-selling opportunities and improved customer retention rates. Personalized services, informed by accurate classifications, lead to higher customer satisfaction and loyalty.
Generative AI
Generative AI is emerging as a key capability in modern financial services, particularly in areas where content generation, automation, and personalization intersect. Institutions are beginning to integrate these models not just for experimentation, but to support core functions like client engagement and operational reporting.
In customer experience, generative models such as GPT-4 are being used to craft tailored communication based on behavioral data, financial activity, and individual preferences. This level of personalization at scale was previously out of reach.
Firms now deliver dynamic investment summaries, personalized offers, and contextual support in digital banking channels. Trinetix reports that leading banks are using generative AI to increase conversion through real-time, automated content that aligns more precisely with user profiles.
On the operational side, financial reporting is undergoing a significant shift. Deloitte has documented examples of firms automating management and regulatory reporting with generative AI, allowing teams to translate complex data into accurate, audit-ready narratives within minutes. This reduces the dependency on manual data synthesis and improves consistency across reports.

From a strategic perspective, the gains are considerable. Research from McKinsey estimates that generative AI could improve global sales productivity in financial services by 3 to 5 percent, with even greater long-term potential across knowledge-intensive roles. These gains are not theoretical. Early adopters are already documenting improvements in output quality, time savings, and employee focus on higher-value tasks.
Generative AI is not a future technology waiting for validation. It is becoming a competitive factor in how financial institutions deliver value, both to their clients and within their internal operations.
Predictive AI
Predictive AI has become an indispensable tool in the financial sector, enabling institutions to anticipate market trends, assess risks, and make informed decisions. By analyzing vast datasets, predictive models can forecast future outcomes with remarkable accuracy, enhancing strategic planning and operational efficiency.
In the realm of investment management, predictive AI algorithms analyze historical market data, economic indicators, and geopolitical events to forecast asset price movements. This empowers portfolio managers to optimize asset allocation and identify lucrative investment opportunities. For instance, a study highlights how predictive analytics can drive enhanced decision-making in finance, allowing organizations to plan effectively amidst market volatility.
Risk management is another area where predictive AI excels. By evaluating credit histories, transaction patterns, and behavioral data, AI models can predict the likelihood of loan defaults or fraudulent activities. This proactive approach enables financial institutions to mitigate risks before they materialize. Latest reports highlight how predictive analytics helps finance professionals forecast cash flows and manage risks effectively.
Furthermore, predictive AI enhances customer relationship management. By anticipating customer needs and preferences, banks can offer personalized products and services, improving customer satisfaction and loyalty. A survey by Citizens Bank indicates that CFOs are increasingly leveraging AI for customer support and cash flow forecasting, reflecting its growing importance in financial operations.
In summary, predictive AI empowers financial institutions to navigate the complexities of the modern financial landscape with greater confidence and agility. By harnessing the power of data-driven insights, organizations can enhance decision-making, mitigate risks, and deliver superior value to their stakeholders.
Key use cases in financial services
AI is actively reshaping core functions inside financial institutions. Whether it's compliance, fraud detection, or customer engagement, the real-world applications are where the business value becomes clear.
Mitigating risk and managing compliance
Financial institutions are increasingly leveraging AI to enhance compliance and risk management. At JPMorgan Chase, AI has improved operational efficiencies and reduced paperwork for both employees and regulators, particularly in fraud detection, where it has significantly reduced false positives.
Regulatory technology (RegTech), powered by AI, automates compliance monitoring by scanning financial transactions, communications, and contracts for potential violations, thereby reducing manual workload and minimizing human error.
However, adoption of AI in risk and compliance is still in its early stages. A survey found that only 5% of respondents predict widespread adoption of AI in risk and compliance within the next 12 months, highlighting a cautious approach in the industry.
Preventing fraud and financial crime
AI has become a critical tool in combating financial fraud. According to a recent industry survey, 74% of financial institutions reported using AI for financial crime detection, and 73% for fraud detection. Despite this widespread adoption, all respondents expected financial crime and fraud activity to rise in 2024, highlighting the continued need for investment in AI solutions.
The effectiveness of AI in fraud detection is evident, with businesses integrating robust AI fraud detection tools seeing up to a 40% improvement in fraud detection accuracy, helping reduce false positives and enhancing security measures.
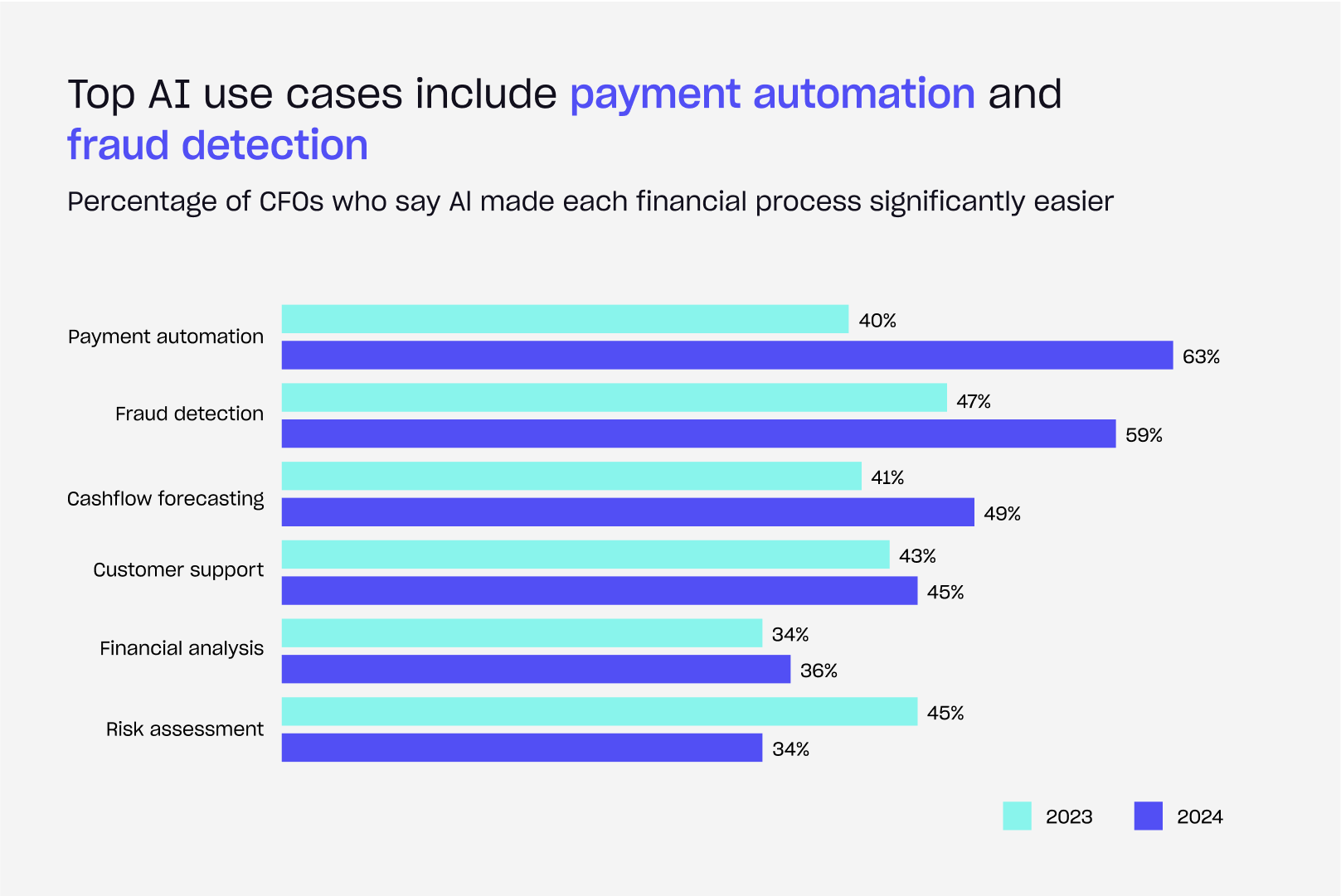
Furthermore, the AI in the fraud detection market is projected to grow significantly, from USD 12.1 billion in 2023 to USD 108.3 billion by 2033, at a CAGR of 24.5%, underscoring the increasing reliance on AI to combat fraud.
Improving customer experiences
AI is also transforming customer service in the financial sector. In practice, companies like Fiserv have integrated conversational AI to enhance their customer feedback process. By using Qualtrics conversational intelligence, Fiserv transformed traditional surveys into dynamic, two-way conversations, leading to a 10-point increase in Net Promoter Scores (NPS) across several customer touchpoints.
Additionally, AI-powered chatbots in finance are streamlining customer experiences by providing real-time assistance, reducing response times, and handling routine inquiries, thereby allowing human agents to focus on more complex issues.
Over time, these tools help build stronger customer relationships and foster brand loyalty in an increasingly competitive market. Moreover, by analyzing interactions at scale, AI enables continuous service improvement, helping financial institutions anticipate customer needs and proactively offer relevant solutions.
Building an AI-native financial future
Artificial intelligence is reshaping financial services at the foundation. It's no longer confined to isolated use cases. It now plays a defining role in how institutions operate, compete, and evolve. The shift is not just technological, it’s strategic.
But unlocking the real value of AI requires more than adopting new tools. It demands robust data, coordinated processes, and a thoughtful integration of human oversight. Institutions that lead with AI do more than automate. They redesign how decisions are made, how risks are managed, and how customers are served.
Looking ahead, the next wave of innovation will bring deeper intelligence, greater personalization, and systems that can learn and adapt faster than ever before. The challenge now is to move with intent—building systems that are resilient, responsible, and ready to scale.
At Aubergine, we help financial organizations do exactly that. Our teams bring together strategy, design, and engineering to craft AI-native digital products that are intelligent, intuitive, and built to perform. We work closely with visionary leaders to translate bold ideas into meaningful impact.
If you're ready to create the next generation of financial experiences, let’s build it together.