Software as a Service (SaaS) has undergone several transformations since its inception in the late 1990s, from on-premise to cloud, from desktop to mobile, from monolithic to microservices. Today, we're witnessing perhaps the most profound evolution yet: the AI transformation of SaaS.
Recent market trends paint a compelling picture. Analysts project rapid growth in the AI-powered SaaS segment, driven by increasing enterprise investment in automation, data intelligence, and decision-making at scale. A growing number of SaaS companies now list AI capabilities as central to their product strategy as no longer optional, but foundational to competitiveness. What we're experiencing is not merely a technology upgrade cycle, but a fundamental evolution.
We've rapidly evolved from systems that offered descriptive analytics to those providing predictive insights and now to prescriptive guidance. The insights here explore how AI is shaping SaaS from the ground up and how stakeholders can prepare for this wave of evolution.
State of AI in SaaS 2025
Artificial intelligence is reshaping the foundations of SaaS. What began as isolated features is now driving end-to-end product transformation. Software is becoming context-aware, continuously adaptive, and capable of taking meaningful action without waiting for user input.
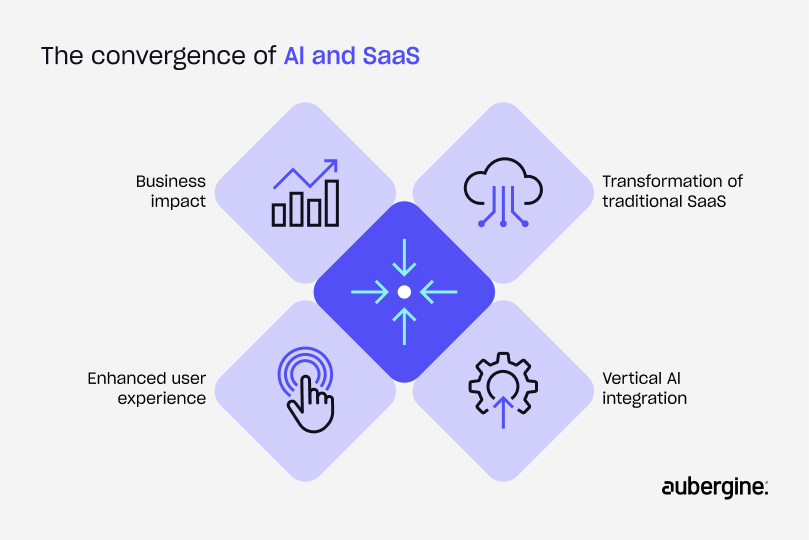
This shift is redefining user experience, architecture, and business strategy. Interfaces are evolving from static forms to intelligent flows. Customer support is being rebuilt as an ecosystem of agents that resolve issues autonomously. Data strategy is moving beyond storage and retrieval to structured, semantic understanding. Even pricing is changing, reflecting value generated by AI rather than access alone.
As AI and SaaS converge, companies are rethinking how products are built, how users engage, and how intelligence is delivered at scale.
Below, we outline some of the most profound ways in which AI is shaping the SaaS ecosystem in 2025.
Operational intelligence & data-driven decisions
Modern AI-powered SaaS platforms process data in real-time, enabling personalization at an unprecedented scale. For example, a marketing automation platform no longer just segments audiences based on historical data, but predicts customer behavior, dynamically adjusts messaging based on real-time signals, and continuously optimizes for outcomes rather than just activities.
Similarly, intelligent automation is transforming complex workflows. Consider accounts payable: platforms like Stamplior Tipalti now use AI to automatically extract invoice data, validate it against purchase orders, detect anomalies, approve payments within policy thresholds, and escalate only true exceptions for human review. These systems continuously learn from each transaction to improve accuracy, speed, and fraud detection over time.
Perhaps most remarkably, these platforms evolve as they’re used. The software you rely on Monday may have subtly improved by Friday, shaped by how you and thousands of others interact with it, creating a feedback loop that drives smarter performance without manual tuning.
Re-defining the SaaS experience
The SaaS experience is no longer defined by dashboards and drop-downs. AI agents are now transforming how users interact with software, shifting from manual control to autonomous action. Instead of asking software to perform tasks, users are increasingly supported by agents that proactively analyze data, make decisions, and take action in real time.
This shift is already underway in insurance. Companies like Tractable use AI to assess vehicle damage from photos and automate claims decisions in minutes, dramatically reducing time to resolution.
Lemonade deploys AI agents like "Jim" to handle everything from policy creation to claims payouts without human involvement. The experience for customers becomes instant and personalized. Filing a claim is triggered by a single tap or can even be automatically initiated based on weather data and policy risk profiles.
For insurers, these agents detect fraud, flag anomalies, and streamline entire workflows. Adjusters are notified only when human judgment is required, not to chase down data or initiate reviews. The result is reduced overhead and improved accuracy.
This new model of SaaS is deeply integrated, context-aware, and autonomous. Interfaces are conversational but also adaptive, capable of learning individual workflows and adjusting in real time. The goal is no longer usability but invisibility. Software fades into the background while value delivery comes to the foreground.
The business model is evolving just as rapidly. SaaS companies are moving away from charging for features and toward pricing tied to outcomes. If an AI agent prevents fraud, saves operational costs, or generates revenue, its value is directly measurable, and billing can reflect that.
SaaS vendors now compete on agent intelligence, vertical expertise, and the strength of their real-time data pipelines. In this new landscape, the winner is not the one with the most tools, but the one whose software thinks and acts with the most precision.
Automation in SaaS operations
Modern systems are moving beyond automating individual tasks to orchestrating entire business processes. They're connecting across applications with semantic understanding rather than brittle point-to-point integrations. And they're smart enough to know when to work autonomously and when to bring in human expertise.
This philosophy extends to resource management, where AI is transforming SaaS economics. Machine learning models now predict usage patterns with remarkable accuracy, dynamically allocating resources where needed.
A healthcare system comprising 12 hospitals utilized AI-powered infrastructure analytics to streamline their data center operations. By consolidating resources and migrating workloads to the cloud, they achieved a 40% reduction in physical servers, resulting in $12.3 million in savings over three years.
Even software development itself is being transformed. AI now generates test scenarios, creates boilerplate code, and even implements self-healing capabilities that can reduce downtime by up to 70%. For development teams, this means faster cycles and the ability to focus on high-value innovation rather than routine maintenance.
Elevating customer service
Customer service is no longer a cost center, but a strategic advantage. AI-led SaaS development is at the heart of this shift, turning reactive support models into intelligent, proactive systems that drive customer success. In 2025, 80% of companies are either using or planning to adopt AI-powered chatbots for customer service, underscoring the shift towards AI-driven support models.
This transformation is happening across the SaaS landscape. Yesterday's rule-based chatbots have evolved into contextually aware support systems that understand intent, recognize emotions, and maintain conversation context. Along with answering questions, they also anticipate needs.
The most innovative companies have completely reimagined where support happens. Rather than sending users to a separate help center, intelligent assistants now appear within the application itself, understanding exactly what you're trying to accomplish and providing guidance in the moment.
What's truly revolutionary is the shift from reactive to proactive support. Modern systems analyze usage patterns to identify potential problems before users even notice them. When they spot multiple customers struggling with the same feature, they alert product teams to potential usability issues.
These capabilities extend beyond traditional support to transform the entire customer journey:
- Predictive models identify at-risk accounts before traditional metrics would detect problems
- Personalized education adapts to each user's role and learning style
- AI-powered insights turn vendors from support providers to strategic advisors
The result? Customer relationships that focus on business outcomes rather than troubleshooting features
The rise of Agentic AI and Action Models
SaaS is entering a new era where software no longer just responds to users, but acts on their behalf. AI agents are evolving into core operational layers, executing tasks, managing workflows, and making decisions with minimal human involvement. This shift has led to the emergence of AI Agents as part of the core SaaS offering, a model where companies subscribe to autonomous agents that deliver outcomes as the core service.

Agentic AI is already transforming the way teams work. Instead of clicking through interfaces or submitting forms, users rely on agents that operate across platforms, connect through APIs, and carry out tasks based on context and goals. In many cases, the agent, not the user, is the one navigating the SaaS environment.
Consider these examples:
- Aisera integrates with systems like Salesforce and ServiceNow to automatically resolve IT tickets, HR questions, and customer service issues.
- Bardeen performs cross-tool automations, pulling data from websites and moving it between Notion, Google Sheets, and CRMs without manual input.
- Tavus generates personalized sales videos by combining CRM data with generative AI, replacing the need for human video production at scale.
Modern SaaS companies represent a growing class of AI agents capable of operating across multiple platforms, learning from interactions, and improving over time. The impact of this shift is being felt across the SaaS stack. Agents often interact directly with systems through APIs, bypassing traditional UIs altogether. This is changing how SaaS is designed and consumed:
- Platforms are being built with agent access in mind, prioritizing modular APIs and data availability.
- Companies are adopting value-based pricing, charging for measurable outcomes like tasks completed, time saved, or results delivered.
- Product strategy is shifting from feature development to agent enablement.
But with increased autonomy comes the need for control. SaaS companies exploring AI must implement strong governance.
It’s important to recognise the scale of this shift. This is not a shift in user interface but a transformation in the nature of SaaS itself. Teams are no longer operating software directly. They are subscribing to intelligent agentic platforms that act on their behalf, predict outcomes, deliver results, and evolve with use.
Intelligent SaaS needs a new tech stack
While building an AI-driven compliance platform for a fintech client who has since raised Series A funding and been recognized for social impact, we were looking for systematic solutions to a classic problem: their legacy stack couldn’t support what AI models needed to do.
Traditional SaaS infrastructure wasn’t built for AI. The shift to intelligent software demands a complete overhaul, from compute to integration:
Infrastructure is evolving rapidly. Machine learning workloads demand specialized resources like GPUs and TPUs instead of general-purpose computing. The most competitive companies are forging strategic partnerships with hardware providers to gain performance and economic advantages their competitors can't match.
Processing location matters more than ever. Some operations need the power of centralized cloud resources; others benefit from edge processing to reduce latency or address privacy concerns. The most innovative systems distribute computation optimally across this spectrum.
Data architecture is being completely reimagined. AI-powered knowledge graphs are replacing traditional data lakes and warehouses, representing not just data points but the relationships between them. Real-time processing has become non-negotiable, and synthetic data solves challenges from privacy concerns to training edge cases.
Perhaps most transformative is the evolution in how systems integrate. Modern APIs don't just exchange data but expose machine learning capabilities directly. Semantic integration layers understand the meaning of information across applications, enabling much more sophisticated workflows while raising important questions about data governance.
The companies that win won't just be those with the best algorithms. They'll be the ones who rebuilt their foundations for this new reality.
From strategy to execution: Leading the AI transformation
For leaders navigating this landscape, three priorities emerge:
- Make strategic technology bets. Will you build proprietary AI capabilities, partner with specialized providers, or leverage open-source foundations? Each path has profound implications for your competitive position.
- Reimagine your product roadmap. The most successful companies are shifting from feature-based plans to outcome-focused objectives, maintaining flexibility about the specific AI capabilities that will deliver those outcomes.
- Develop new organizational capabilities. Beyond technical skills like machine learning and prompt engineering, teams need deep expertise in data governance, ethical AI, and the regulatory landscape.
At Aubergine, we help startups and legacy businesses alike meet this moment. Whether you're integrating AI into existing systems or building intelligent products from scratch, we design and deploy workflows that scale, align with your values, and drive meaningful outcomes.
From rapid prototyping to enterprise-grade implementation, we partner to make AI real while building ethically, strategically, and at speed.